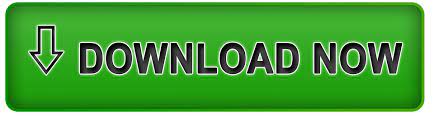

The advantage of a one-pass clustering strategy is that it saves on the computational time required to analyze the sequences in the provided study. UNOISE3 uses a one-pass clustering strategy that does not depend on quality scores, but rather two parameters with pre-set values that were curated by its author to generate “zero-radius OTUs” ( Edgar, 2016). Deblur employs a sample-by-sample approach which reduces both memory requirements and computational demand. Deblur aligns sequences together into “sub-OTUs” and, based on an upper error rate bound along with a constant probability of indels and the mean read error rate, removes predicted error-derived reads from neighboring sequences ( Amir et al., 2017). This approach is advantageous as it builds unique error models for each sequencing run.

DADA2 generates a parametric error model that is trained on the entire sequencing run and then applies that model to correct and collapse the sequence errors into what the authors call amplicon sequence variants (ASVs) ( Callahan et al., 2016). These pipelines differ in how they correct sequencing errors.
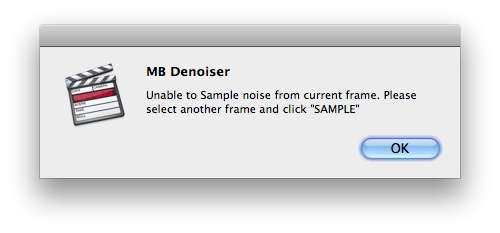
Recently, many new bioinformatic sequence “denoising” approaches have been developed to address this issue by attempting to correct sequencing errors thus improving taxonomic resolution. To avoid this issue sequences are often clustered into operational taxonomic units (OTUs) at a particular identity threshold (e.g., 97%) to avoid the problem of differentiating biological from technical sequence variations however, this comes at the cost of taxonomic resolution. However, sequencing errors make it difficult to distinguish biologically real nucleotide differences in 16S sequences from sequencing artefacts. This sequencing approach is often used to avoid the high cost of shotgun metagenomic sequencing or to avoid problems with sequencing non-microbial DNA from host contamination. The 16S rRNA gene (16S) is usually chosen as a marker gene for sequencing of bacterial communities due to its unique structure that contains both conserved and variable regions and its presence in all known Bacteria and Archaea species. Microbiome studies often use an amplicon sequencing approach where a single genomic region is sequenced at a sufficient depth to provide relative abundance profiles of the microbes present in a sample. Our findings indicate that, although all pipelines result in similar general community structure, the number of ASVs/OTUs and resulting alpha-diversity metrics varies considerably and should be considered when attempting to identify rare organisms from possible background noise. The three denoising approaches were significantly different in their run times, with UNOISE3 running greater than 1,200 and 15 times faster than DADA2 and Deblur, respectively. The open-reference OTU clustering approach identified considerably more OTUs in comparison to the number of ASVs from the denoising pipelines in all datasets tested. DADA2 tended to find more ASVs than the other two denoising pipelines when analyzing both the real soil data and two other host-associated datasets, suggesting that it could be better at finding rare organisms, but at the expense of possible false positives. Our analysis on real datasets using recommended settings for each denoising pipeline also showed that the three packages were consistent in their per-sample compositions, resulting in only minor differences based on weighted UniFrac and Bray–Curtis dissimilarity. We found from the mock community analyses that although they produced similar microbial compositions based on relative abundance, the approaches identified vastly different numbers of ASVs that significantly impact alpha diversity metrics. In this study, we conduct a thorough comparison of three of the most widely-used denoising packages (DADA2, UNOISE3, and Deblur) as well as an open-reference 97% OTU clustering pipeline on mock, soil, and host-associated communities. As more researchers begin to use high resolution ASVs, there is a need for an in-depth and unbiased comparison of these novel “denoising” pipelines. However, there have been numerous bioinformatic packages recently released that attempt to correct sequencing errors to determine real biological sequences at single nucleotide resolution by generating amplicon sequence variants (ASVs). Traditionally, sequence reads are clustered into operational taxonomic units (OTUs) at a defined identity threshold to avoid sequencing errors generating spurious taxonomic units. High-depth sequencing of universal marker genes such as the 16S rRNA gene is a common strategy to profile microbial communities.
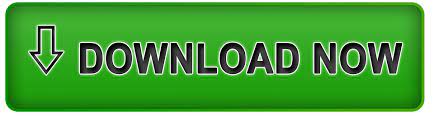